The Very Large Array Sky Survey (VLASS) is creating a map of nearly 80% of the radio sky. As part of this survey, more than 10 million new radio sources will be detected. It would take a tremendous amount of time to verify all those sources so Dr. Brian R. Kent, a scientist at the National Radio Astronomy Observatory, is developing a way to use neural networks to automate the process. Using software such as Keras and TensorFlow, Brian trained a neural network to classify radio sources found in VLASS data.
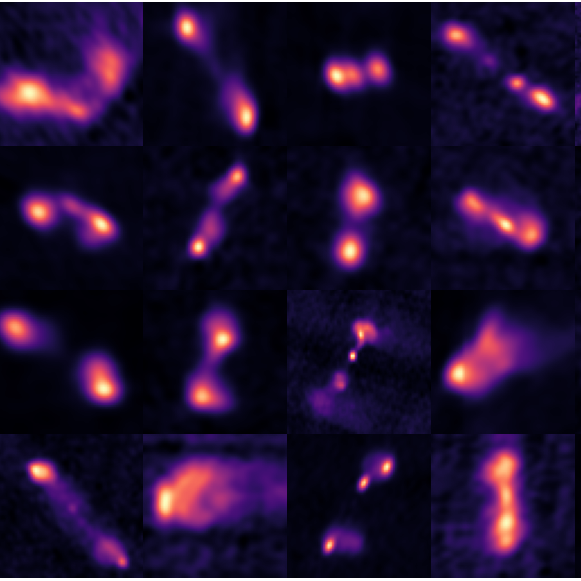
Most VLASS radio sources are Active Galactic Nuclei (AGNs). They often appear as points with radio lobes on either side, which are the radio signals created by the jets of supermassive black holes. Once trained on known radio sources, Brian’s neural network was able to identify these sources effectively. But interestingly, it also sometimes identified an unusual source: something that resembled an AGN, but looked a bit strange. The neural network isn’t efficient at detecting these strange sources, but the ones it finds are worth further investigation.
Thanks to this work, astronomers will not only have a detailed catalog of AGN radio sources, they will also have some interesting new radio sources with plenty of mysteries waiting to be explored.